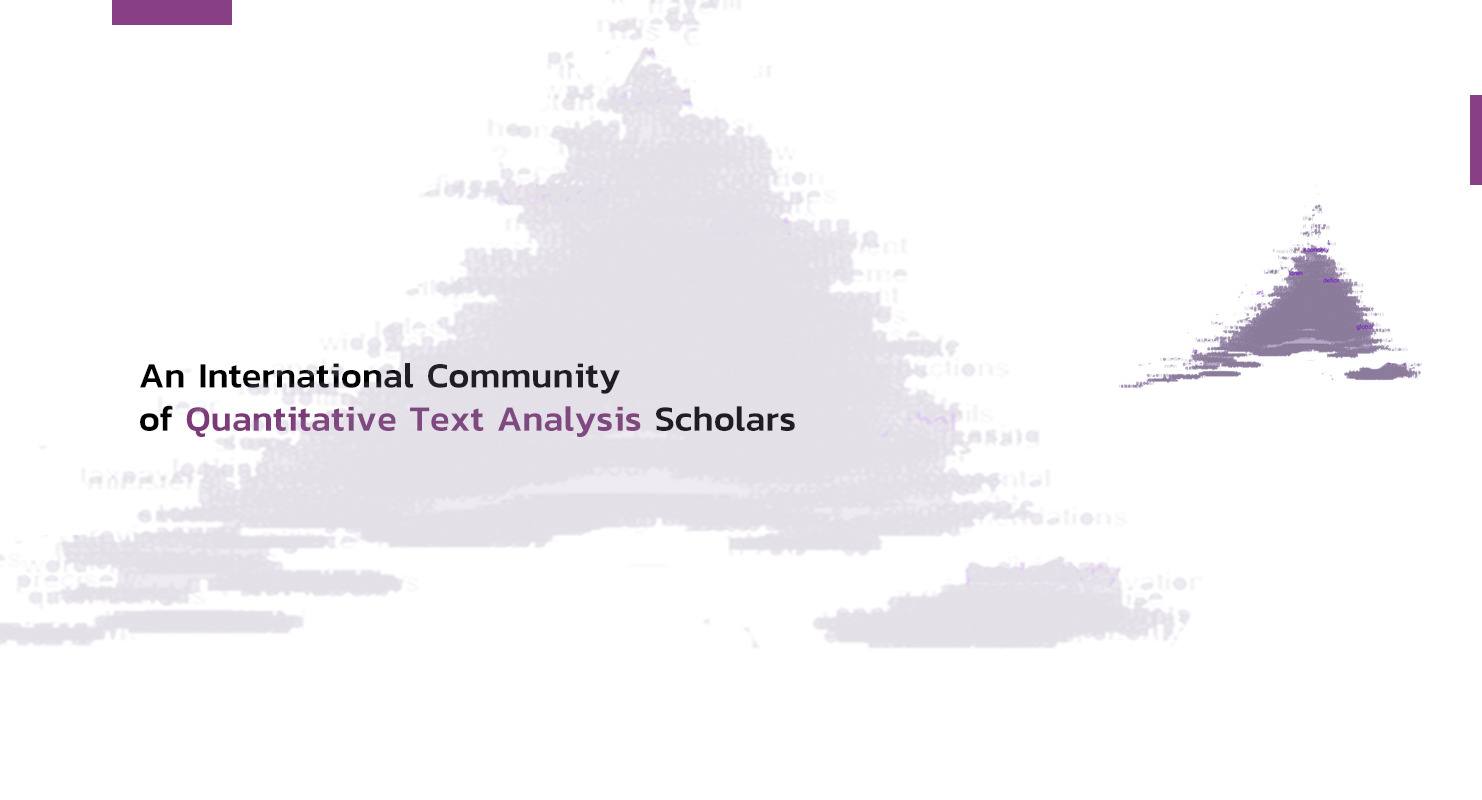
Tutorial – 9:30am CET
Quantitative Text Analysis for Absolute Beginners: (Stefan Müller & Kenneth Benoit)
About the tutorial:
The workshop provides a hands-on introduction to quantitative text analysis using quanteda (https://quanteda.io) and related R packages. In the first part of the workshop, participants will first learn and apply how to import texts in various formats into R. Afterwards, we describe the functionality of text corpus, explain the difference between types and tokens, and reshape the level of texts from documents to sentences or paragraphs. Afterwards, we turn to tokenization and discuss ways of selecting, removing tokens as well as detecting and compounding multi-word expressions. Finally, we will construct a document-feature matrix for the quantitative analysis of textual data.
The applied elements of the workshop will make use of the R programming language. Prior knowledge of text analysis is not required. Participants without any prior experience with R are encouraged to read Chapter 1 of the quanteda tutorials (https://tutorials.quanteda.io)
The second part of the workshop “Quantitative Text Analysis for Absolute Beginners” provides an overview of textual statistics, such as readability, text similarity, keyness, and lexical diversity. Moreover, participants will get to know and apply textual scaling models, such as Wordscores and Wordfish. The last part of the tutorial introduced supervised machine learning which leverages human coding to classify large amounts of unlabelled texts.
The applied elements of the workshop will make use of the R programming language. Prior knowledge of text analysis is not required. Participants without any prior experience with R are encouraged to read Chapter 1 of the quanteda tutorials (https://tutorials.quanteda.io). We strongly recommend attending both sessions of the workshop “Quantitative Text Analysis for Absolute Beginners”.
Stefan Müller is an Assistant Professor and Ad Astra Fellow in the School of Politics and International Relations at University College Dublin. He is a founding member of the Connected_Politics Lab at University College Dublin, core contributor to the quanteda R package, and Training Advisor of the Quanteda Initiative CIC. Stefan’s research focuses on the interactions between political parties, voters, and the media. He develops and validates user-friendly tools for the efficient and reliable combination of human coding and machine learning.
Kenneth Benoit is Professor of Computational Social Science in the Department of Methodology at the London School of Economics and Political Science. His current research focuses on computational, quantitative methods for processing large amounts of textual data, mainly political texts and social media. Kenneth Benoit is the creator of the quanteda R package and Managing Director and Founder of the Quanteda Initiative CIC.